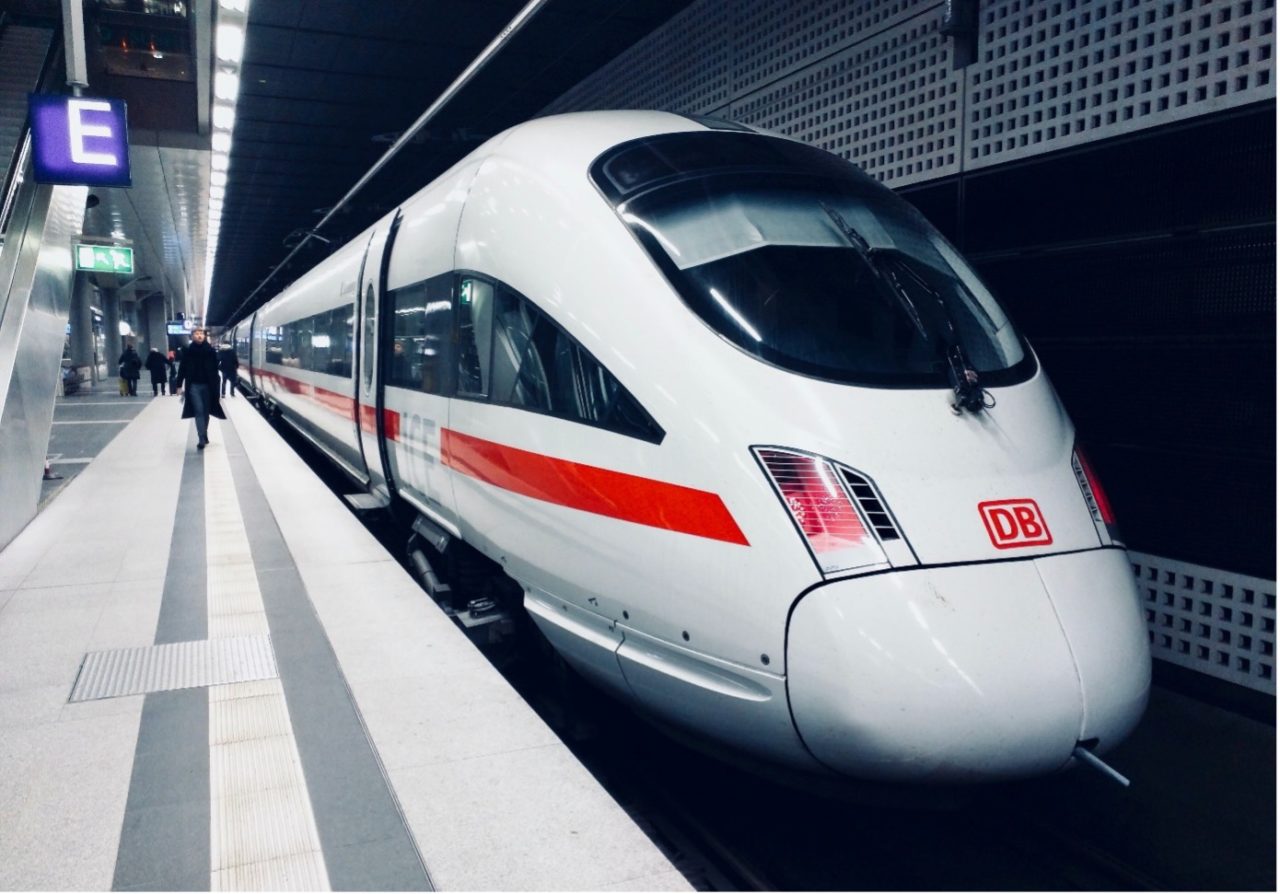
How much are common machine learning classifiers, such as Random Forest (RF), Support Vector Machines (SVM), and Artificial Neural Networks (ANN) better in forecasting than traditional discrete choice models? To which extent is it worth to sacrifice the improved interpretability of the latter to achieve a higher prediction accuracy (PA)?
As part of a project with Deutsche Bahn (DB), we investigated these issues using three high-dimensional, longitudinal (on average, 80 choices per construction site) datasets on train route scheduling with more than 200,000 choice observations, 39 explanatory features and five choice alternatives. The models were trained on the 2020 dataset and validated for 2021 and 2022. Importantly, our validation approach is twofold conservative, since we do not just predict on new construction sites, but also on completely new planning horizons – given the basic requirement of creating the construction schedule on an annual basis.
While RF performs best in terms of PA (2021: 60.8%; 2022: 58.6%), the improvements of about 6%-points compared to the Mixed Logit model are modest. Results indicate that a substantial amount of unobserved construction site heterogeneity is present, which RF cannot capture either. ANN and SVM performed notably worse than the RF approach, but still better than the discrete choice models. The moderate performance of the ANN and SVM relative to the RF model may be related to an improved ability of the latter to capture non-linear relationships and higher-order interactions. While ANN and SVM may shine when it comes to pattern recognition, image processing, speech or signal decryption, their benefits are limited in the current application, also in terms of computation time.
Basil Schmid studied economics at the University of Zurich and received his PhD from ETH Zurich at the Institute of Transport Planning and Systems (IVT) in 2019. Currently, as a senior assistant at IVT, he is responsible for numerous projects, including the new Swiss Value of Time Study (VSS), the ETH Mobility Initiative, a project with Deutsche Bahn on modeling train control decisions, and an app-based GPS tracking study on time use (SNF). He has published in numerous journals such as Transportation Research Part A, Journal of Choice Modeling, and Transportation, and is co-developer of the R package mixl for estimating complex decision models.