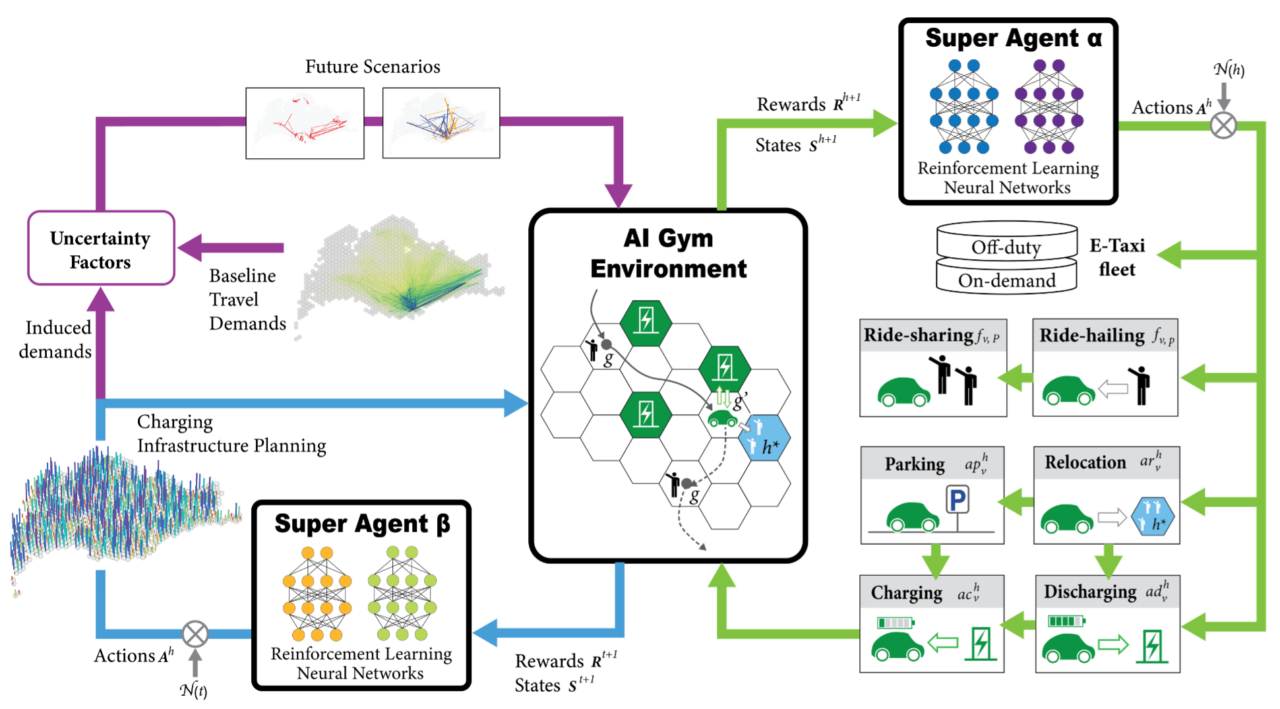
Although a transition to electric vehicles (EVs) has potential to greatly reduce transportation-related greenhouse gas emissions, it faces significant challenges, including range anxiety and limitations in battery capacity. Effective long-term planning for EV charging infrastructure can overcome these barriers and increase the chances of widespread adoption.
The authors propose a multi-period planning approach for this effective long-term planning that uses exploration of many future scenarios of transport system evolution and reinforcement learning. The approach is based on the idea that planners update their understanding of the evolution of the transport system over time as they optimally sequence decisions with respect to the location, size, and types of chargers. This approach supports the adaptive planning of the built environment and thereby has the potential to enable planners to better reach societal goals.
Background and Problem Statement
Transportation contributes 15% of the world’s greenhouse gas emissions, playing a major role in climate change [1]. Transitioning to EVs can contribute greatly to meeting climate goals [2]. The primary barriers facing a transition to EVs are the limitations in battery capacity, and the corresponding range anxiety concerned by consumers [3]. Developing an appropriate charging infrastructure system can greatly help to mitigate range anxiety and enhance EV adoption. As the creation of such a system cannot happen instantaneously, long-term planning is required. And as long-term planning is faced with consideration uncertainties as to how the future will unfold, planners must strategically determine the optimal number, types, and locations of charging stations to meet both current and future EV charging demands over time. As planning charging infrastructure over a long horizon is a sequential decision problem in which there are considerably and growing uncertainties over time, planners must decide the location, size, and types of chargers, in each planning period, considering constraints such as land availability, technological readiness, and annual budgets, as well as how the system has changed in the previous periods.
Challenges
More specifically, there are three challenges to be overcome in the long-term planning of charging infrastructure. First, accurately forecasting charging demand trajectories and their spatial-temporal patterns is difficult. Conventional prediction methods often oversimplify demand growth, ignoring the induced demand from new infrastructure. Second, predicting the technological readiness of charging technologies and their acceptance by the public is also challenging. Third, long-term land availability is unpredictable, adding another layer of uncertainty and constraint to infrastructure placement.
Approach
The approach proposed by the authors is illustrated in the Figure above. It includes three main modules. First, The demand estimation module (purple loop) simulates annual variations in travel and charging demands, incorporating a feedback loop to account for induced EV charging demands. Second, The traffic simulation module (green loop) modelled the detailed dynamics of EV charging behaviours and other operations, capturing the operational performances of charging infrastructure. The intervals of updating system states for this module is second. Third, the charging infrastructure planning module (blue loop) suggests to planners the most suitable location, size, and types of charging infrastructure.
Qiming Ye is a postdoctoral researcher and the module coordinator of the Adaptive Mobility Infrastructure and Land-use (AMIL) module at Future Cities Laboratory Global, at the Singapore ETH Centre. Holding a PhD in Transport Engineering from Imperial College London, his research pursues the development of transport-specific AI tools (i.e., reinforcement learning methods) for the dynamic control of intelligent transport systems and the adaptive planning of transport infrastructure under stochastic conditions for the long term.
Prof. Dr. Bryan T. Adey is the head of the Infrastructure Management Group in the Institute of Construction and Infrastructure Management at the D-BAUG, Swiss Federal Institute of Technology in Zürich (ETH Zürich). His research is focused on improving the effectiveness and efficiency of infrastructure management. This includes the definition and standardization of the infrastructure management process, from setting goals to determining optimal monitoring and intervention programmes, and evaluating the performance of infrastructure management organisations. It also includes the automation of parts of the infrastructure management process, e.g. the determination of optimal intervention strategies for single infrastructure objects and the development of algorithms to generate network intervention plans that maximise net benefit. Other themes of his research are estimating the risk related to infrastructure, including estimating infrastructure behaviour when subject to extreme events, estimating the resilience of the infrastructure and the system in which it is embedded, and estimating the optimal ways to restore infrastructure following extreme events. Prof. Adey works on infrastructure management issues related to many types of infrastructure, including road networks, rail networks, water distribution networks, sewer networks and building portfolios.
Dr Prateek Bansal is a Presidential Young (Assistant) Professor who joined the Department of Civil and Environmental Engineering in December 2021. Before joining NUS, he was a Leverhulme Trust Early Career Fellow at Imperial College London. Dr Bansal leads the Behavioural Cognitive Science (BeCoS) lab at NUS. His group develops novel methods at the intersection of AI, Econometrics, Causal Inference, and Psychology to nudge and forecast mobility behaviour and the adoption of emerging technologies at an individual level and urban scale.