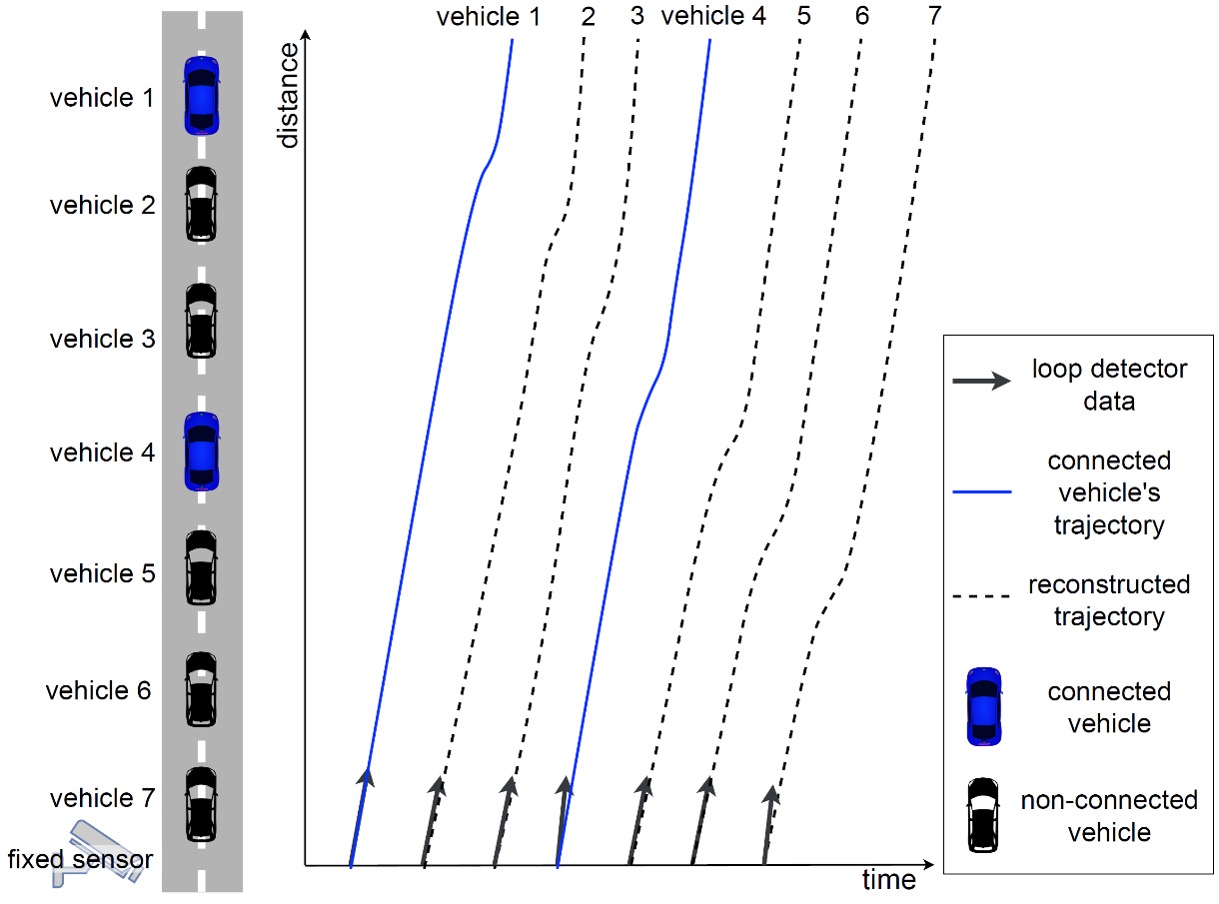
Understanding how vehicles move is crucial for safer, more coordinated, and smarter transportation systems. However, directly observing vehicle trajectories is impractical, posing a challenge to obtaining accurate trajectory data with limited sensing capabilities. We explore our innovative approach to vehicle trajectory reconstruction, reshaping the future of traffic management.
Understanding how vehicles move is crucial for many reasons—like predicting traffic congestion, reducing energy consumption, and keeping traffic flowing smoothly. But to do this, we need to know exactly where each vehicle is, and that’s where things start to get tricky. So, how do we solve this puzzle? That’s what we’re here to crack.
Some methods rely on fixed sensors like loop detectors, cameras, and radars to monitor passing vehicles. These sensors give us helpful details, including how many vehicles are on the road and how fast they’re zipping along, which helps us estimate traffic speed and flow. But they don’t tell a full story of trajectories, so we need a smarter approach.
That’s where connected vehicles swoop in. They feed us real-time updates on their whereabouts, helping us grasp driving patterns, foresee traffic snarls, and keep tabs on vehicles. But even as we look towards a future where all cars are linked up, we’re still dealing with a mix of connected and unconnected vehicles for the next few years.
Our magic solution? Mixing data from connected vehicles with speed intel from loop detectors. This combo helps us grasp how traffic waves move using both sets of data. Picture this: when one vehicle slows down on the highway, it sets off a chain reaction. Vehicles trailing behind also hit the brakes, creating a wave of sluggish traffic, also known as a shockwave. Understanding how these waves roll helps us piece together the trajectory of vehicles. As a result, we can infer the trajectory of a stretch using trajectory data from a limited number of connected vehicles, since the trajectory of different vehicles affected by the same traffic shockwave remains the same.
We’re zooming in on the speed at which these shockwaves spread, since it greatly impacts how the wave moves through traffic, affecting the accuracy of our trajectory reconstruction. We focus on how to nail down reasonable values for the shockwave speed using mixed and limited sensing data. A Monte-Carlo Sampling-based method is carefully designed to solve the shockwave speed value and is further applied to reconstruct the trajectory of the non-connected vehicles.
We’re honing in on highways to test our proposed method. By blending different data types and understanding how traffic shockwaves flow, we’re able to piece together vehicle trajectories with precision. So, hop on board as we cruise towards a future where managing traffic isn’t just simpler—it’s way cooler.
The related paper was recently accepted by Expert Systems with Applications and can be found here.
Yifan Zhang is a Postdoctoral Researcher at the Traffic Engineering group (SVT) of the Institute for Transport Planning and Systems (IVT), ETH Zürich. Her research interests include intelligent transportation systems, autonomous vehicles, and microscopic traffic modeling.