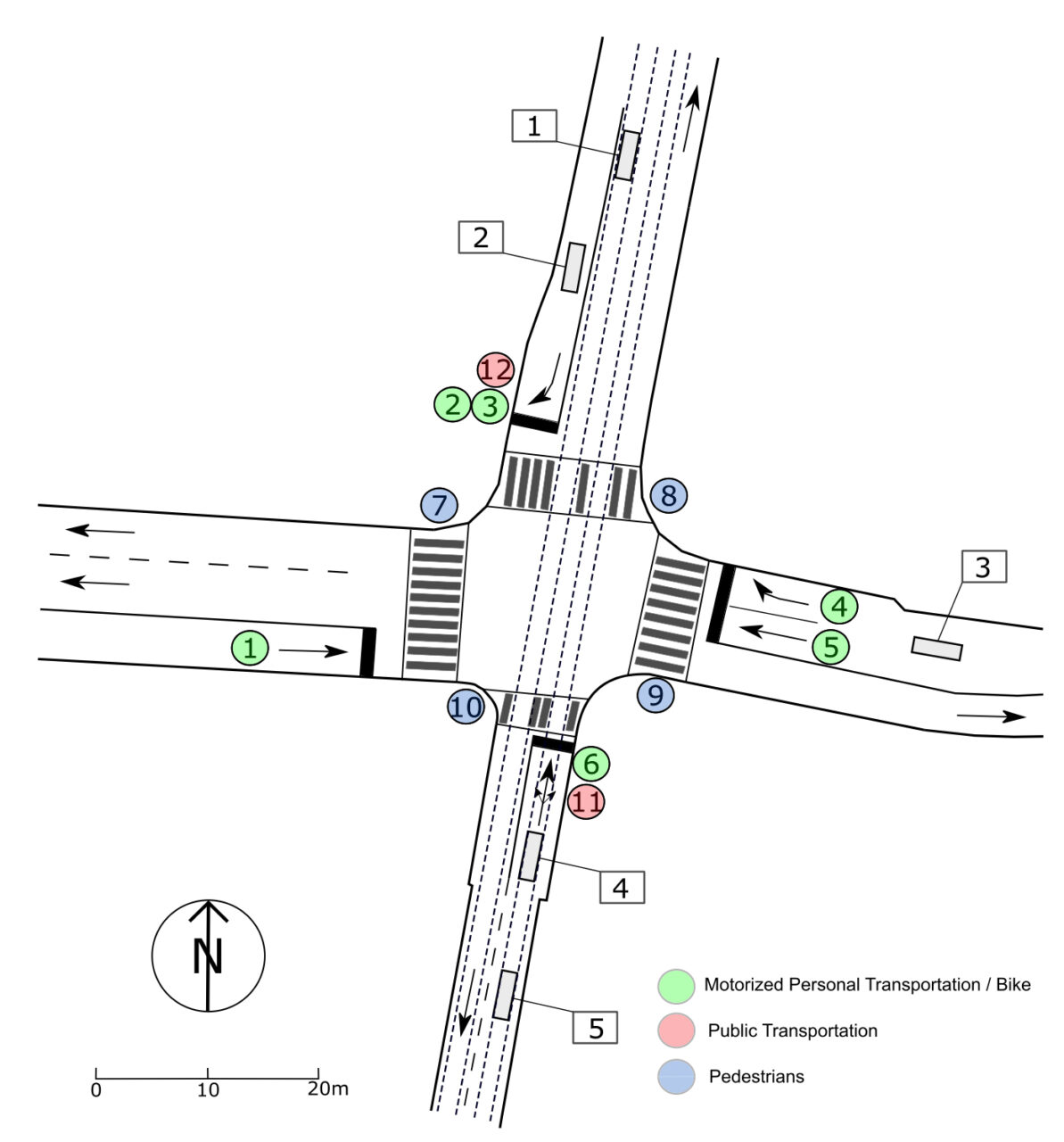
Alexander Genser, Michail A. Makridis, Kaidi Yang, Lukas Ambühl, Monica Menendez, Anastasios Kouvelas
2024
Full text
Recently, efforts have been made to standardize signal phase and timing (SPaT) messages. These messages contain signal phase timings of all signalized intersection approaches.
This information can thus be used for efficient motion planning, resulting in more homogeneous traffic flows and uniform speed profiles. Despite efforts to provide robust predictions for semi- actuated signal control systems, predicting signal phase timings for fully-actuated controls remains challenging. This paper pro- poses a time series prediction framework using aggregated traffic signal and loop detector data. We utilize state-of-the-art machine learning models to predict future signal phases’ duration. The performance of a Linear Regression (LR), Random Forest (RF), a light gradient-boosting machine (LightGBM), a bidirectional Long-Short-Term-Memory neural network (BiLSTM) and a Temporal Convolutional Network (TCOV) are assessed against a naive baseline model. Results based on an empirical data set from a fully-actuated signal control system in Zurich, Switzerland, show that state of the art machine learning models outperform conventional prediction methods.
Citation: Genser, Alexander, et al. «Time-to-Green predictions for fully-actuated signal control systems with supervised learning.» IEEE Transactions on Intelligent Transportation Systems (2024).