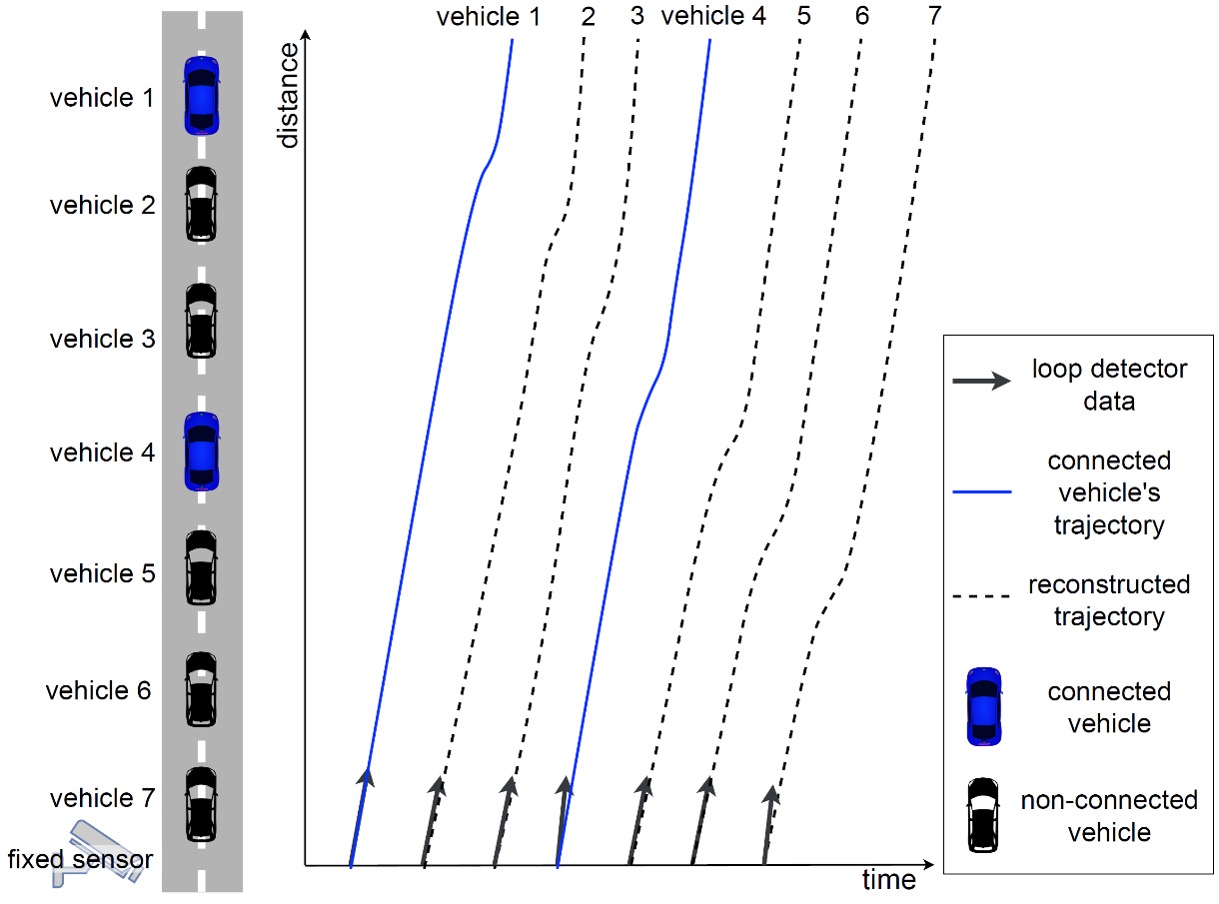
Yifan Zhang, Anastasios Kouvelas, Michail A. Makridis
2024
Inference of detailed vehicle trajectories is crucial for applications such as traffic flow modeling, energy consumption estimation, and traffic flow optimization. Static sensors can provide only aggregated information, posing challenges in reconstructing individual vehicle trajectories.
Shockwave theory is used to reproduce oscillations that occur between sensors. However, as the emerging of connected vehicles grows, probe data offers significant opportunities for more precise trajectory reconstruction. Existing methods rely on Eulerian observations (e.g., data from static sensors) and Lagrangian observations (e.g., data from probe vehicles) incorporating shock-wave theory and car-following modeling. Despite these advancements, a prevalent issue lies in the static assignment of shockwave speed, which may not be able to reflect the traffic oscillations in a short time period caused by varying response times and vehicle dynamics. Moreover, energy consumption estimation is largely ignored. In response, this paper proposes a novel framework that integrates Eulerian and Lagrangian observations for trajectory reconstruction. The approach introduces a calibration algorithm for time-varying shockwave speed. The calibrated shockwave speed of the CV is then utilized for trajectory reconstruction of other non-connected vehicles based on shockwave theory. Additionally, vehicle and driver dynamics are introduced to optimize the trajectory and estimate energy consumption. The proposed method is evaluated using real- world datasets, demonstrating superior performance in terms of trajectory accuracy, reproducing traffic oscillations, and estimating energy consumption.